Integrated Process Modelling – Very Useful Bioprocess Digital Twin
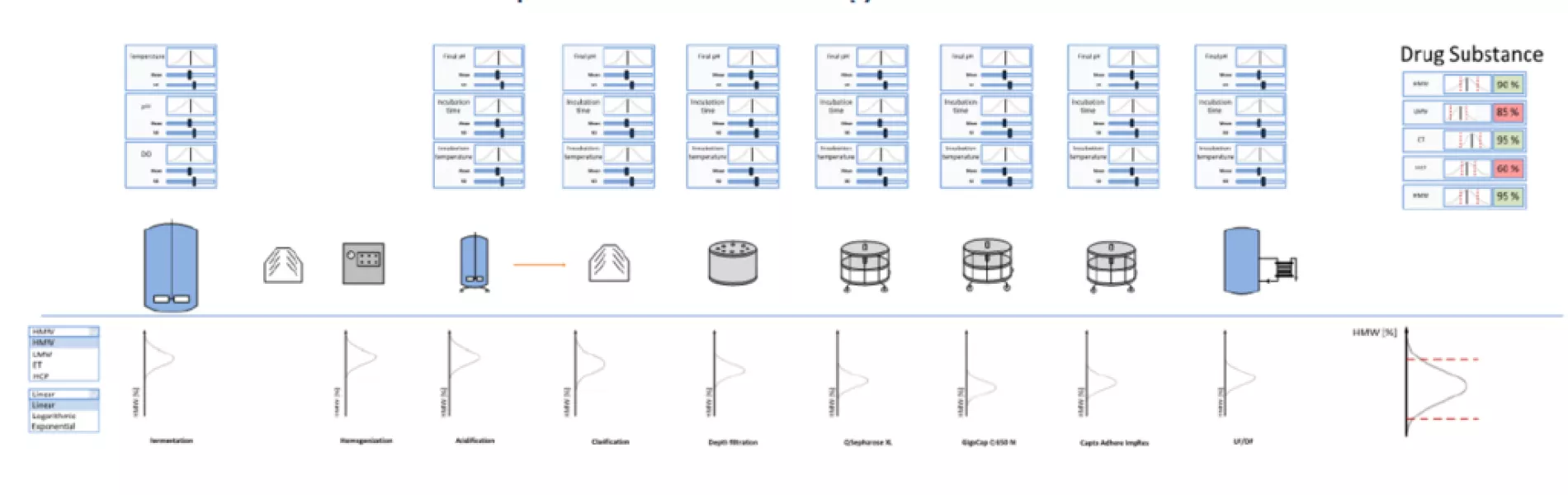
The concept of the digital twin sounds like science fiction: a complete digital representation of your process or system, along with all the flexibility to play with simulations, creating feedback, and targeting optimizations. However, the foundation for such fantastical notions are already well established in many industries, and increasingly, in Bioprocessing as well.1 ,2
The idea is not completely new: in 2016, Colin Harris, VP of Software Research at GE presented a tool for industrial processes that was positively fascinating. Colin Harris interacted with a voice-activated software to remotely mitigate damages to a wind turbine on the other side of the world. He addressed the software as “Twin” and the two calmly saved millions of dollars within seconds. This is the future, right? Yes, but there are a lot of steps to develop in current modelling techniques and simulations before the technology becomes the standard. And we must come to terms with how the application of this technology changes the way we do bioprocess development, process validation, and GMP manufacturing.
What is a Bioprocess Digital Twin?
It would be only a slight exaggeration to say the digital twin is a full process-assistant who has access to all historical data and present process conditions, and who can simulate the future based on all active models and settings.
- 1Kroll, P., et al., Workflow to set up substantial target-oriented mechanistic process models in bioprocess engineering. Process Biochemistry, 2017. 62: p. 24-36.
- 2V. Haunschmid, T. Natschläger. Enabling automated model maintenance by application-oriented model diagnostic measures, APACT 2018 - Advances in Process Analytics and Control Technology 2018 Conference (2018)

In more detail, the bioprocess digital twin is a comprehensive, integrated mathematical model of the complete process chain. It runs in real-time or in batch mode, incorporates development and current manufacturing data. It’s models train on PAT data, quality data, and time-series SCADA data. They make use of merged ODE, PDE, MLR and bayesian statistical models. And they can predict critical quality attributes and key performance indicators throughout the process chain.
Concretely, the digital twin’s inner workings are a concatenation of all available models, (mechanistic or statistic), in their correct process-sequence order. At Exputec, we refer to this as the “Integrated Process Model (IPM).” Attached to the IPM, is the ability to simulate the process within estimated variation in order to gain access to enormous amount of output data without having to perform a single run at scale.
What is the application of a Bioprocess Digital Twin?
The build-up of the digital twin, while a triumph by itself, is actually only the first step. The benefits are derived only with the applications stemming from the availability of this centralized model. The following sections depict the four principle pillars of bioprocessing digital twin applications:
1) Digital twins cut costs in bioprocess development
Starting with the very first data in early process development, the presence of a digital twin can drastically alter the industrialization pathway. The twin may explore early design space, suggesting further experimental designs, and then training on the new data iteratively. This leads both to reduced experimental workload as well as well as deeper knowledge of the design space. Additionally, using applications such as parameter sensitivity analysis, risk assessments can become a data-driven exercise, based on thousands of simulations within a judged range, removing some of the subjectivity inherent in risk management.
2) Digital twins reduce effort in setting up a control strategy
Process validation was one of the first published applications of digital twins. In process characterization studies, data driven setting of parameter normal operating ranges (NORs) and proven acceptable ranges (PARs) can be implemented, based on a holistic look at the impact by the process parameters on the attributes throughout the process chain. Simulating through a variety of parameter settings, it is possible to derive exactly where the attribute is likeliest to be out-of-spec at the end of the process, regardless of how early in the process we are performing the evaluation.
3) Digital twins accelerate process performance qualifications
During process performance qualification (PPQ), biopharma companies calculate the necessary number of PPQ batches. The digital twin simulations will increase confidence, even while reducing the number of required PPQ runs.
4) Digital twins reduce out of spec events and supports problem solving in manufacturing
The digital twin simulations will predict Bulk Substrate and Finished Product specification variation better than individual unit operation models. With these simulations, precise acceptance criteria and control limits can be estimated not only for the intermediates, but for the end results. This will result in less deviations and OOSs.
Additionally, the twin can lead to faster problem solving, when OOSs do occur: atypical outcomes can be traced back through the simulations to investigate potential special cause variation, whether univariate or multivariate.
What do you need to build and run a digital twin?
Bioprocess digital twins leverage data from process development data, designs of experiment, manufacturing data, mechanistic modelling, and even from risk assessments. Therefore, a scalable, asset-centric data foundation for both manufacturing and development data is critical. To build digital bioprocess twins, asset connectivity is required, as is statistical analytics, and mechanistic modelling, all in one environment. This should include data management capabilities linking data sources from LIMS, ELNs and MES, such as time value pairs, 2D and 3D ,with above mentioned algorithms for analyzing uncertainties and its propagation along the process chain.
Is it worth to go for it?
The considerations required for building up a digital twin are not for the weak-hearted. There are numerous assumptions and hard decisions required to accurately depict a process entirely in a digital manner. Thus, the digital twin is always wrong, but sometimes helpful. Also, its development never finished and need to be adapted continuously along the product life cycle along ICH Q12 principles. Hence, we do not encourage to invest into tailor made models for specific products only. They will only be useful for blockbuster products, but may eventually die, when its developer has focused on other tasks. We suggest using generic workflows for target oriented digital twins (Kroll et al. 2017) and automated model management (Haunschmid, 2018), which can run as SaaS solution in the cloud. This will the future for quick assembly and maintenance of the digital twins on a methodological platform, independent of the modelling “nurd”. Like this, the Return of Investment is almost immediate, focussing process characterization work, giving specifications for intermediate acceptance criteria, reducing the number of experiments of linkage studies, identifying variations during tech transfer.
Hence, the benefits are clear and digital twins represent the future towards the streamlining and organizing of process knowledge within central models.
We don’t yet speak with digital twins to solve our bioprocessing tasks, as Dr. Harris did. However, the movement in this direction is a bright sign for the future, and it will be to the advantage of the industry to leverage these advances.